This post explains how to drawn the exact equation of the locus of maximum rates for an exothermic reversible reaction.
Model
We define the simple following reaction:
With rate is defined as:
Where the thermodynamic and kinetic constants and
are modeled as:
Defining the conversion ratio , concentrations can be expressed as
assuming there is no product B at the beginning. Then we can state:
Where is the equilibrium conversion ratio for a given temperature
. This equation is the envelope of all other curves as it is impossible to go beyond the equilibrium.
Similarly, reaction rate can be written in term of :
And isolate for a given rate
:
For an exothermic equilibrium those curves are concave (negative convexity) and therefore exhibits maximum. Putting and solving for
, we get the following expression:
Which is the locus of maximum rates (optimal temperature pathway) for the given reaction.
Symbolic check
We can check out the math using sympy
:
import sympy as sp
R = sp.Symbol("R", positive=True)
T = sp.Symbol("T", positive=True)
DrH = sp.Symbol("\Delta_{R}H^0")
x = sp.Symbol("x", postive=True)
xe = sp.Symbol("x_e")
K0 = sp.Symbol("K_0")
Ea = sp.Symbol("E_a")
k0 = sp.Symbol("k_0")
A0 = sp.Symbol("A_0")
v = sp.Symbol("v")
KT = K0 * sp.exp(- DrH / (R * T))
xe = KT / (1 + KT)
kT = k0 * sp.exp(- Ea / (R * T))
vT = A0 * kT * (1 - x / xe)
xTv = xe * (1 - v / (A0 * kT))
sol = sp.solve(sp.Eq(sp.diff(xTv, T), 0), v)
sol2 = sp.solve(sp.Eq(sol[0], vT), x)
# [E_a*K_0/(E_a*K_0 + E_a*exp(\Delta_{R}H^0/(R*T)) - \Delta_{R}H^0*exp(\Delta_{R}H^0/(R*T)))]
Which is same expression that we derived above.
Numerical example
Lets write a small class that brings the math:
import numpy as np
import matplotlib.pyplot as plt
class Equilibrium:
R = 8.314
def __init__(self, DrH0, K0, Ea, k0, A0=1.):
self.DrH0 = DrH0
self.K0 = K0
self.Ea = Ea
self.k0 = k0
self.A0 = A0
def K(self, T):
return self.K0 * np.exp(- self.DrH0 / (self.R * T))
def xe(self, T):
return self.K(T) / (1. + self.K(T))
def k(self, T):
return self.k0 * np.exp(- self.Ea / (self.R * T))
def v(self, T, x):
return self.A0 * self.k(T) * (1. - x / self.xe(T))
def x_otp(self, T):
return self.Ea * self.K(T) / (self.Ea - self.DrH0 + self.Ea * self.K(T))
And create a real world example:
model = Equilibrium(-75300, 0.18955e-10, 48721, 530991)
Tlin = np.linspace(250, 500, 500)
vlevels = np.logspace(-6, -1, 15)
xlin = np.linspace(0., 1., 500)
T, X = np.meshgrid(Tlin, xlin)
xisov = model.v(T, X)
fig, axe = plt.subplots()
c = axe.contour(T, X, xisov, vlevels)
axe.plot(Tlin, model.xe(Tlin), linewidth=2.)
axe.plot(Tlin, model.x_otp(Tlin))
Which renders as follow:
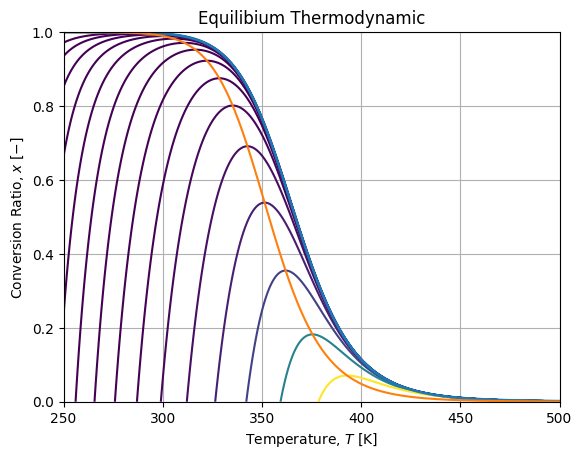
Where the blue curve is the equilibrium envelope, the orange curve is the locus of maximum rates.